«The shameless exaggerations surrounding large language models (LLMs) would be laughable if LLMs were not diverting so much energy, money, and talent into a seemingly bottomless pit.»
– Professor Gary Smith, 2025 (1)
«LLMs have basically indexed the internet, and in processing this data have created links between datasets based on fixed rulesets. This means that similar information is grouped together, weighted and linked to other similar areas. A big mesh of interconnected data. This is not AI. This is data mining.»
– Ian Venner, 2025 (2)
«GenAI models are trained to fill in the blanks. To claim that they do more than this would be an extraordinary claim that should require extraordinary evidence. Instead, we are mostly treated to the logical fallacy of affirming the consequent. The models perform as if they are reasoning (for example), so they must be reasoning. The alternative hypothesis is that they are copying (approximately) the language that was used by reasoning humans.»
– Herbert Roitblat, 2024 (3)
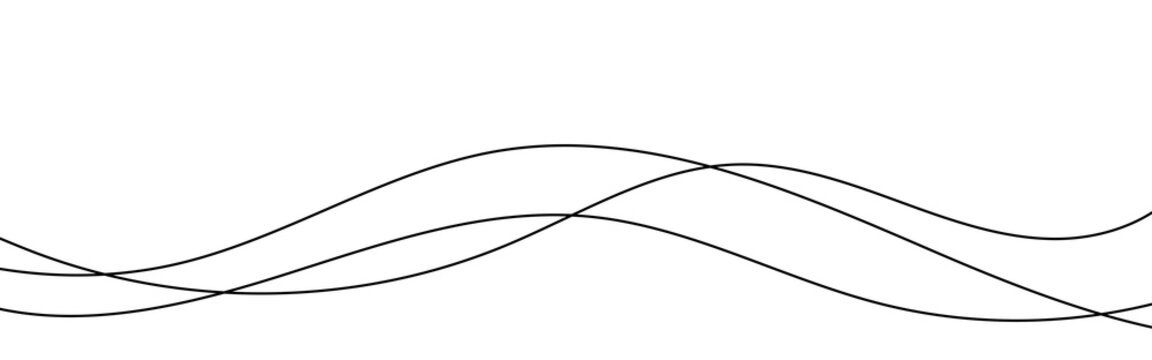
Below is a concise overview of this blog book’s chapters generated by Microsoft Copilot, using its «Think Deeper» option. Copilot’s statistical analysis of the letters and sentences across the various chapters is by no means poor, offering a clear and accessible overview of this book and its chapters.

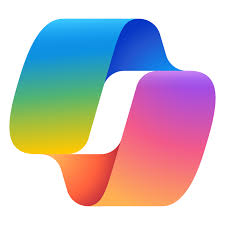
This blog book is focusing on the capabilities, limitations, and implications of modern AI, particularly large language models like ChatGPT and Microsoft Copilot :
- Prologue:
It introduces the theme by linking AI criticism with academic skepticism. The author recounts extensive testing of various chatbots over several years and sets the stage for a detailed exploration of AI’s overhyped reputation and its real limitations . - Chapter 1:
This chapter argues that while AI chatbots such as ChatGPT can mimic human language, they fundamentally operate on statistical pattern recognition—not genuine intelligence. It questions the appropriateness of the term “artificial intelligence,” suggesting that “conversation robots” is a more accurate label . - Chapter 2:
The discussion here centers on common misconceptions. It stresses that despite advanced outputs, these systems do not “understand” input in any human sense; they simply utilize machine learning algorithms, and true general AI remains a distant prospect . - Chapter 3:
Through various tests, the chapter reveals that ChatGPT and similar models often generate responses that are superficially convincing but lack depth and accuracy, especially when tackling complex academic tasks requiring higher-order thinking . - Chapter 4:
This section delves into the mechanics of LLMs. It compares modern chatbots to earlier systems (like ELIZA), emphasizing that they merely pattern-match rather than engage in critical analysis or reflection. It also highlights issues such as limited memory and susceptibility to misinformation . - Chapter 5:
Focusing on higher-order thinking, the chapter critiques AI-generated texts for their tendency to hallucinate or fabricate information. Even though these systems can produce structured and fluent responses, they falter when deeper analysis and logical consistency are required . - Chapter 6:
Here, the evaluation of multiple AI tools on a challenging academic assignment demonstrates that all tested systems struggle with creative reasoning and problem solving. The chapter underscores the gap between the tools’ evolving capabilities and the demands of academic rigor . - Chapter 7:
The discussion shifts to education, arguing that banning AI tools like ChatGPT is impractical. Instead, it calls for a balanced use that emphasizes ethical integration, critical thinking, and the development of students’ evaluative skills to make informed use of these technologies . - Chapter 8:
This chapter raises multifaceted ethical, legal, and security concerns. It points out the risks—ranging from data privacy and bias in training data to the environmental impact of massive computing resources—and stresses the need for greater transparency and ethical practices in AI development . - Chapter 9:
The focus here is on rethinking assignment design in higher education. To counteract the easy overreliance on AI-generated content, the chapter recommends assignments that demand deep reflection, critical analysis, and authentic personal insight, thereby reinforcing academic integrity . - Chapter 10:
The concluding chapter reiterates that current LLMs function more as sophisticated predictive systems than as repositories of true knowledge. It highlights the gap between the media’s portrayal of AI and its practical, often limited, application—especially in rigorous academic environments . - Epilogue:
Final reflections express persistent skepticism; although AI chatbots can organize and summarize content, they fall short in providing genuine comprehension and complex reasoning. Tests conducted between 2022 and 2025 on tasks from the field of Social Informatics demonstrate that while tools like ChatGPT can craft superficially coherent academic responses, they lack the critical analysis, deep reasoning, and synthesis essential for higher-level work in the Social Sciences and Humanities.
Each chapter builds toward a critical view of today’s AI tools, urging educators, researchers, and policymakers to temper expectations and focus on integrating AI responsibly while acknowledging its current limits.
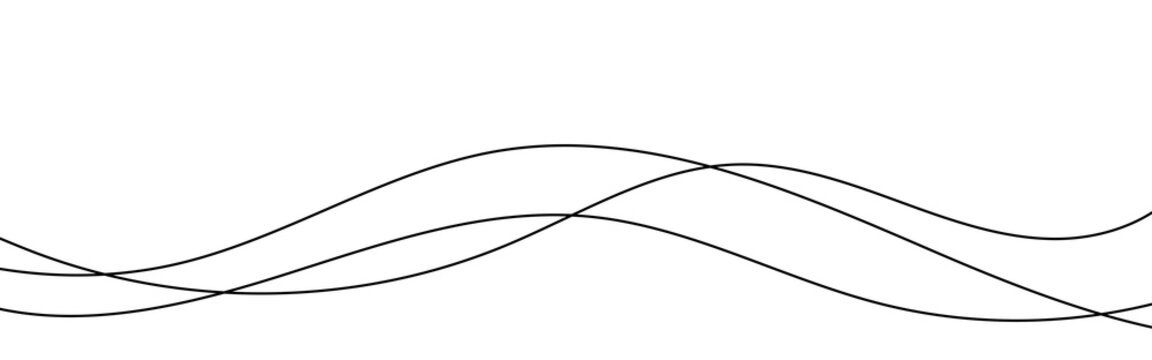